Leila Donn 2019 Field Report
Leila Donn, PhD Student, Department of Geography and the Environment, University of Texas at Austin
Project: New machine-learning computer program to find and study caves, karst, and climate in the Guatemalan tropical forest of the Maya Lowlands
The main objective of my summer 2019 fieldwork was to ground-truth the results of a LiDAR-based machine-learning computer program designed to identify potential cave entrances. The secondary fieldwork objective was to collect a stalagmite climate record, if an appropriate one was identified. I was able to comprehensively address the first objective, but I was not able to address the second objective. My field season was a great success, despite an unexpected change of location immediately before the start of the season. Three days before travel I received notice that our permit to work in Guatemala was severely delayed due to the upcoming presidential election. Therefore, my advisor helped me to quickly get permission to work at the Programme for Belize Biosphere Reserve (PfB) in northwestern Belize and stay at an archaeological field camp inside of the Reserve. I was able to run the already-written computer program over the LiDAR imagery for PfB and quickly generate a map of predicted cave entrance locations for my new study site.
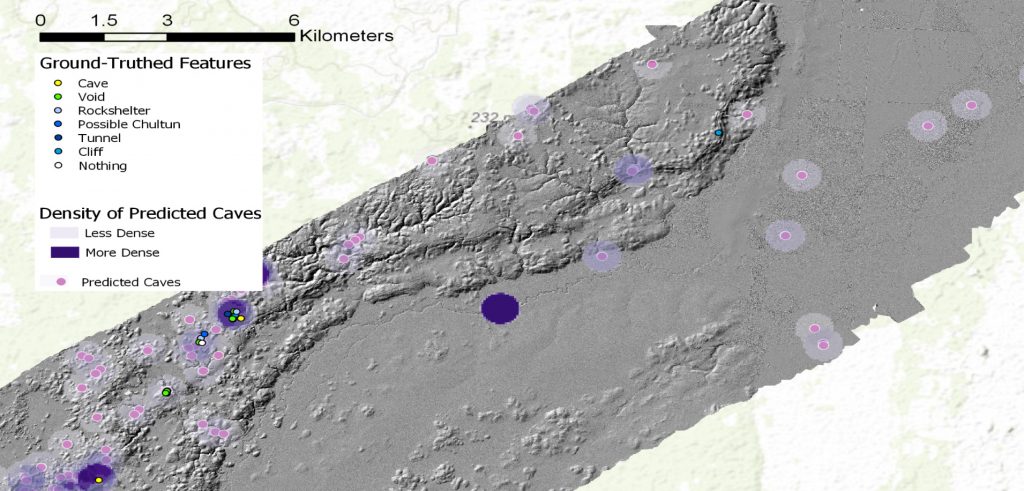
My cave entrance-finding program, which I will hence refer to as ‘The Program,’ enables the efficient identification of caves in hard-to-access areas located under dense tropical forest canopy. The Program identified about 50 potential cave entrances within the approximately 250,000-acre PfB (Figure 1). These individual points were concentrated in four main areas within which the density of potential cave entrances was highest.
One point identified by The Program turned out to be a 30-foot-high x 60-foot-long bedrock cliff that contained several fairly large voids (1.5 ft-long) that terminated too shallowly to be called caves. Over the next few days (6/7-6/10) we ground-verified about 8 cave points, of which 7 were cave-like features. This included 2 rock shelters, 3 of which appeared to be chultuns (underground storage containers constructed by the ancient Maya, usually carved into an existing space in the rock), and 2 voids or holes in rock faces. On 6/11 we found our first true cave (Figure 2), right where one of my Program points predicted. With two days of fieldwork left we found the most impressive cave-like feature identified during the field season: a 200-foot-long x 100-foot-wide x 160-foot-deep collapsed cave complex (a type of sinkhole; Figure 3). The feature was composed of two adjoining sinkholes, connected in the center by a giant arch with a land bridge above it (this was part of the ancient cave roof that had not yet collapsed).
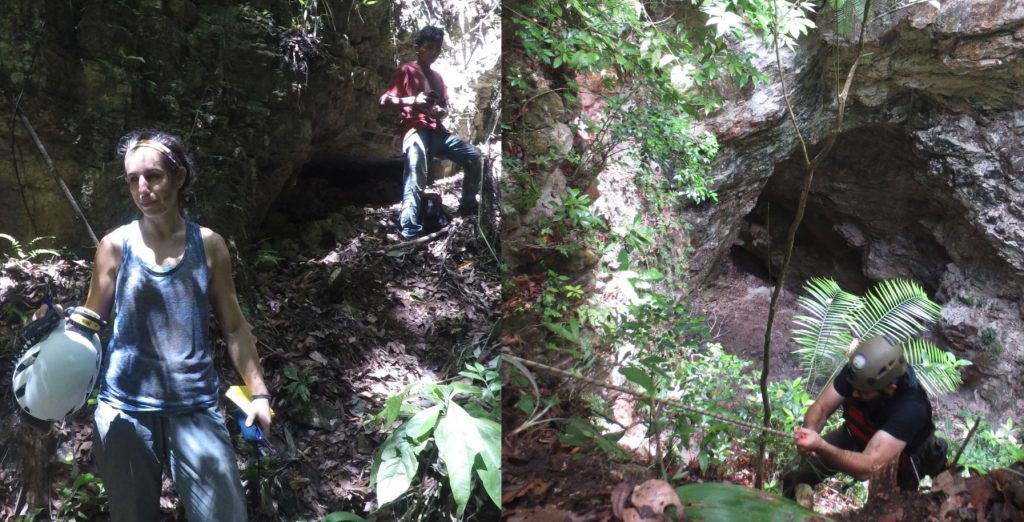
The Conference of Latin American Geography PhD Field Study Award helped make this work possible, The ground-truthing that I completed this summer confirms that my machine-learning cave entrance identification methodology works. It also made it clear that integrating the LiDAR point cloud into the model will help to make it more accurate. Though I did not find any stalagmite climate records, I will continue this portion of the project at two different sites in Guatemala next year. The machine-learning methods that I am developing will be geographically transferable and have the potential to be of service outside of academia across a range of uses such as natural hazard identification (faults, sinkholes, landslides), forest inventory mapping, and planning and development work.
Please see the full report for more details